We're a spatial computing company creating city-scale augmented reality in a real landscape
And it all begins with
a simple phone camera

The AI constantly matches point clouds with the original footage back and forth. This is how we can obtain precise 3D objects even when the camera is moving and the angle of view is changing.
The AI constantly matches point clouds with the original footage back and forth. This is how we can obtain precise 3D objects even when the camera is moving and the angle of view is changing.
.webp)

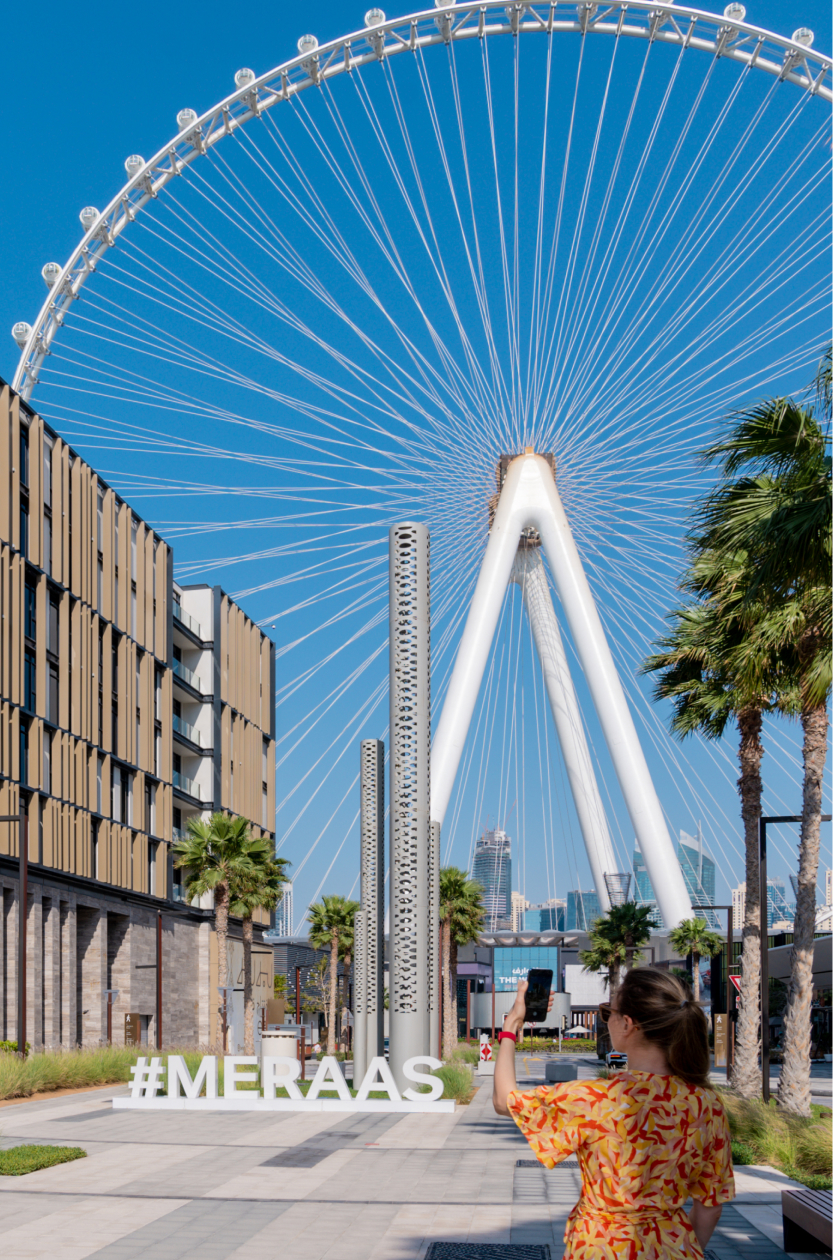
Data Collection
First, we're shooting videos of the location. A smartphone is all it takes to capture the landscape of the environment.

The AI constantly matches point clouds with the original footage back and forth. This is how we can obtain precise 3D objects even when the camera is moving and the angle of view is changing.
The AI constantly matches point clouds with the original footage back and forth. This is how we can obtain precise 3D objects even when the camera is moving and the angle of view is changing.
Processing: Stage 1
Then AI build a 3D-reconstruction. It analyzes video footage frame by frame, identifying key points and transforming it into a spatial representation of the environment.
In other words, algorithms turn flat frames into three-dimensional objects.

The AI constantly matches point clouds with the original footage back and forth. This is how we can obtain precise 3D objects even when the camera is moving and the angle of view is changing.
Processing: Stage 2
Using point clouds, we reconstruct a spatial geometry of the environment—an approximation of it. This digital replica is as close to the real landscape as it can be.

The AI constantly matches point clouds with the original footage back and forth. This is how we can obtain precise 3D objects even when the camera is moving and the angle of view is changing.
Spatial Map
Here, spatial geometry transforms into 3D representation of reality. Now we have the machine-readable format that will be interpreted by the Visual Positioning System (VPS) through algorithms.
This is the final step of landscape digitization and the first step of AR integration.

The AI constantly matches point clouds with the original footage back and forth. This is how we can obtain precise 3D objects even when the camera is moving and the angle of view is changing.
Visual Positioning
Using cues from the footage, VPS locates the position and orientation of the camera within the environment in real time.
It allows us to integrate AR objects into the landscape precisely and seamlessly, making you feel as if you're seeing it with your own eyes.

The AI constantly matches point clouds with the original footage back and forth. This is how we can obtain precise 3D objects even when the camera is moving and the angle of view is changing.
Realistic Augment Reality
With spatial map and VPS, we can integrate virtual objects of any size into any real landscape at any time.
They say the sky is the limit, but not for us.

The AI constantly matches point clouds with the original footage back and forth. This is how we can obtain precise 3D objects even when the camera is moving and the angle of view is changing.
The AI constantly matches point clouds with the original footage back and forth. This is how we can obtain precise 3D objects even when the camera is moving and the angle of view is changing.

The AI constantly matches point clouds with the original footage back and forth. This is how we can obtain precise 3D objects even when the camera is moving and the angle of view is changing.
The AI constantly matches point clouds with the original footage back and forth. This is how we can obtain precise 3D objects even when the camera is moving and the angle of view is changing.
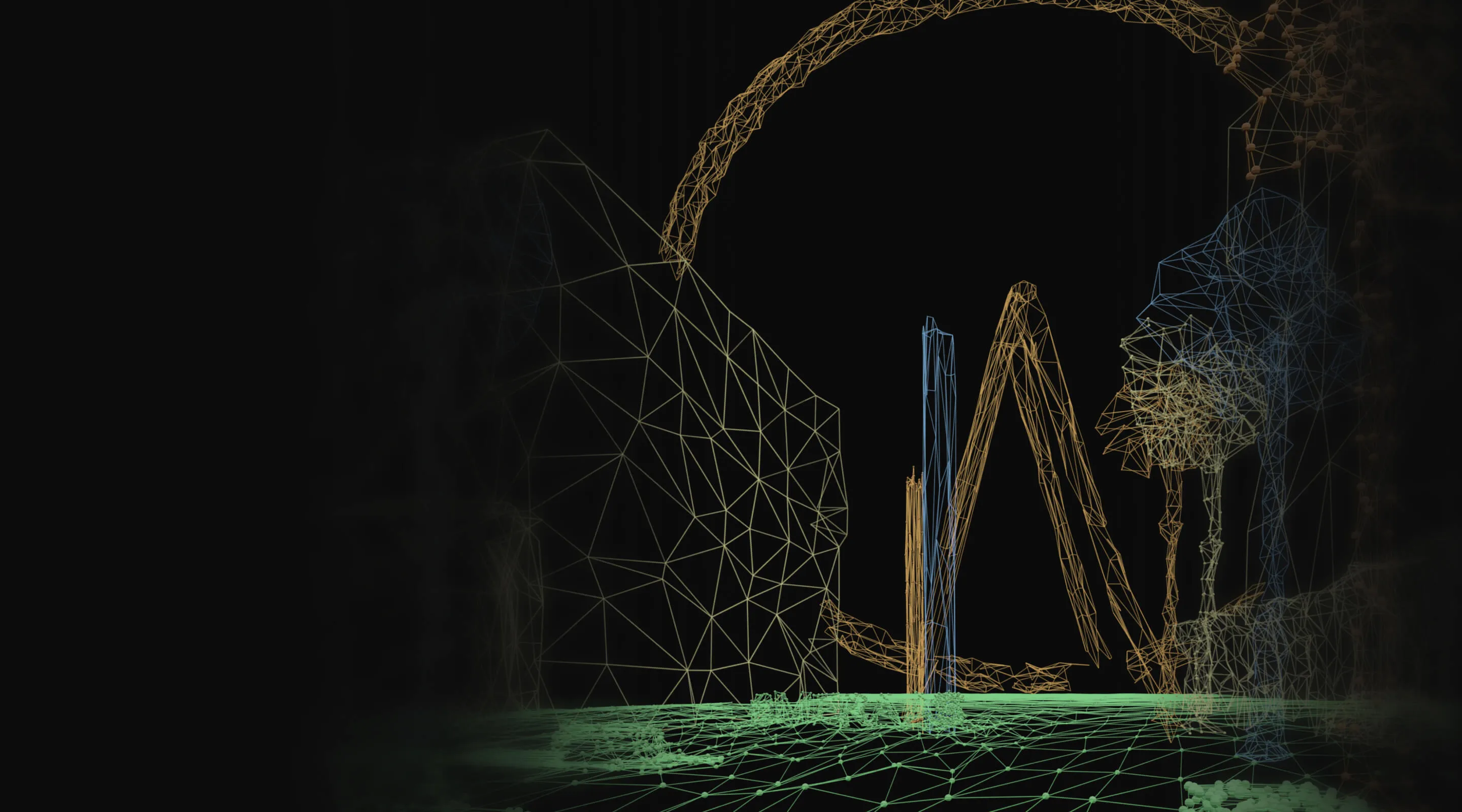
The AI constantly matches point clouds with the original footage back and forth. This is how we can obtain precise 3D objects even when the camera is moving and the angle of view is changing.

The AI constantly matches point clouds with the original footage back and forth. This is how we can obtain precise 3D objects even when the camera is moving and the angle of view is changing.
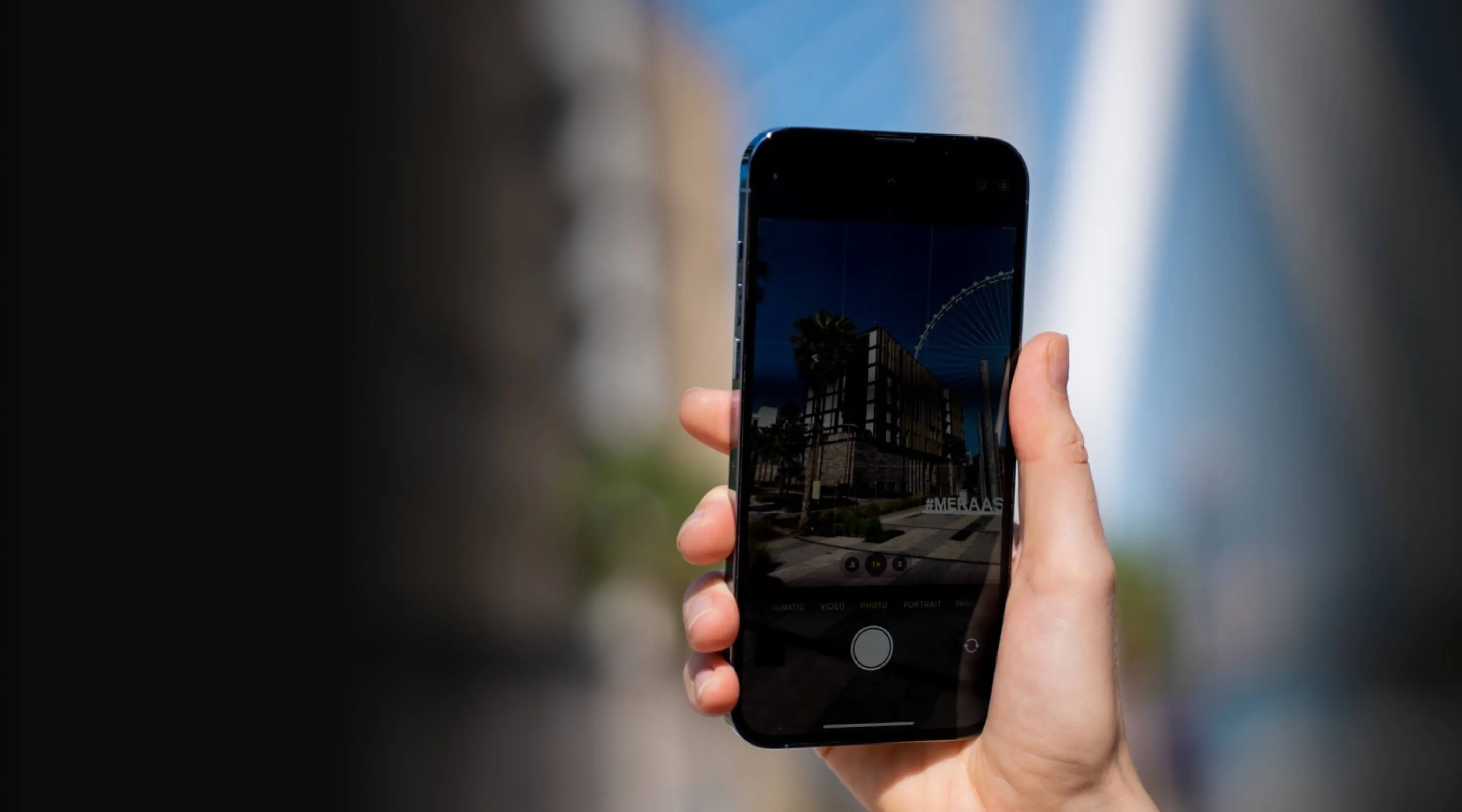
The AI constantly matches point clouds with the original footage back and forth. This is how we can obtain precise 3D objects even when the camera is moving and the angle of view is changing.

The AI constantly matches point clouds with the original footage back and forth. This is how we can obtain precise 3D objects even when the camera is moving and the angle of view is changing.
.webp)
.webp)
.webp)
.webp)
Join Our Team
Explore opportunities and become a part of our journey in spatial computing